Algorithmic randomness and Bayesian convergence to the truthFrancesca Zaffora Blando (Stanford University)
211
Arts West
Parkville 3010
Australia
Organisers:
Topic areas
Details
Francesca Zaffora Blando (Stanford) will present "Algorithmic randomness and Bayesian convergence to the truth" at 11 on 5 April in Arts West 211 West Wing. The talk will be presented remotely.
Abstract: Almost-everywhere theorems abound in mathematics, especially in fields such as analysis, ergodic theory, probability and measure theory. Much recent work in algorithmic randomness has concerned characterisations of randomness notions in terms of the almost-everywhere behaviour of suitably effectivised functions from analysis or probability. In this talk, I will consider Lévy’s Upward Martingale Convergence Theorem from this perspective. The main result I will present is a characterisation of Schnorr randomness in terms of an effectivisation of Lévy’s Upward Theorem.
Besides its intrinsic interest, this result has natural applications for formal epistemology and the philosophical interpretation of probability. In particular, the natural Bayesian interpretation of Lévy’s Upward Theorem is that belief, in the form of an agent’s best estimates of the true value of some integrable random variable, aligns with the truth in the limit almost everywhere. We can think of the random variable as a quantity that the agent is interested in determining: it is fixed once the world is fixed, but it can vary between worlds. We restrict attention to computable random variables because these are the ones whose expectation can be effectively approximated: this constraint thus reflects the restrictions on the agent's epistemic resources. Our characterisation of Schnorr randomness then says that the world is Schnorr random if and only if, for all such computable quantities, the Bayesian agent’s estimate of the quantity at the world eventually aligns with its true value. In this sense, the Schnorr random worlds are exactly the ones in which a certain kind of inductive success is attainable.
I will conclude by briefly discussing analogous characterisations of other standard algorithmic randomness notions. These results illustrate how algorithmic randomness may be used to gauge, via computability-theoretic tools, the success set of computable Bayesian agents in terms of the difficulty of the inductive problems they face. This is joint work with Simon Huttegger and Sean Walsh.Registration
No
Who is attending?
No one has said they will attend yet.
1 person may be attending:
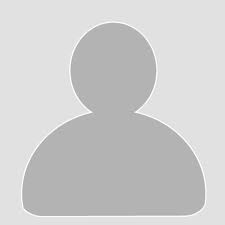
Will you attend this event?